Table Of Content

Then, the cells were washed with PBS for 15 min, fixed with Immunol Staining Fix Solution (Beyotime, P0098) for 30 min and washed with PBS again, followed by treatment with Immunostaining Permeabilization Buffer with Triton X-100 (Beyotime, P0098) for 30 min and washing with PBS again. To stain the lipid droplets, the cells were incubated with Nile Red (2 µM) in the dark for 10–30 min and then washed with PBS before the nuclei were stained with Antifade Mounting Medium with DAPI (Beyotime, P0131). Fluorescence images were captured using an OLYMPUS IX73 fluorescence microscope and Lecia two-photon confocal microscope.
Analysis of the substitution effect of the trifluoromethyl group
The beads were washed 3 times with PBST, and the precipitated proteins were eluted with 1 × SDS loading buffer (Beyotime, L00327) at 100 °C for 5 min and analyzed by Western Blot. Each of the atom features was initially represented as a vector of size 34 using the RDKit python package, and the list of atom features can be found in our previous work. In TransformerCPI2.0, we additionally introduced a new virtual atom that carries the information at the molecular level and does not exist in the given compound. This virtual atom was initialized as the average of atom features across the whole compound and was linked to all atoms. All the atom vectors together with the virtual atom vector were put into GCNs70 to learn the representation by integrating their own neighborhood information. Notably, only one GCN layer was used and recommended in this process, and more than two GCN layers harmed the performance of TransformerCPI2.0 to a great deal.
Programs

Word2vec is an unsupervised technique to learn high-quality distributed vector representations that describe sophisticated syntactic and semantic word relationships and maps discrete words to low-dimensional real-valued vectors. However, the final embedding table of Word2vec is stationary, regardless of the upstream and downstream context information of the given word, which may lead to errors regarding the true meaning of the word in its local context. Since BERT67 achieved great success in natural language processing (NLP), many efforts have been devoted to protein sequence representation learning.
Exploring Nasal Casts in Nasal Drug Development - Aptar
Exploring Nasal Casts in Nasal Drug Development.
Posted: Wed, 24 Apr 2024 16:07:30 GMT [source]
Fluorescence-activated cell sorting (FACS)
It is part of the machine learning method to reduce the risk for a SAR paradox, especially taking into account that only a finite amount of data is available (see also MVUE). Obviously, a relationship exists between the molecular structure of a compound and its activity. The UC DDC was created by the University of California Biomedical Research Acceleration, Integration & Development (UC BRAID) Drugs, Devices, Diagnostics, Development (D4) group, and it has since expanded to actively include industry sponsors. Support for the consortium has been provided by a UC Office of the President (UCOP) Multi-Campus Research Proposal Initiative grant (MRPI) and its industry sponsor(s).
Virtual screening of RNF130
The most closely related proteins in the training data were found to be thyroid hormone receptor β-1 and liver X receptor (LXR) β, sharing a sequence identity of 33% and 30%, respectively, with PPARγ (SI8, Table S8). Benefiting from the end-to-end nature, the sequence-to-drug workflow can be inversely used to enable drug target identification or drug repurposing. This means that we can perform proteome-wide target screening, as only protein sequence information is required except for a given drug molecule as the model input. To date, preclinical and clinical data support the use of PPIs in cancer treatment54, but few new targets have been identified. TransformerCPI2.0 was applied to score 2204 human proteins from the DrugBank database55 against four classic PPIs (rabeprazole, lansoprazole, omeprazole and pantoprazole, Fig. 6a, b, Supplementary Tables 12–15), and the results were sorted by predicted interaction probability. After analyzing the top 20 proteins, ARF1 attracted our attention due to its oncogenic effect on cancer stem cells (CSCs) via the lipolysis pathway56,57.
In addition, a significant accumulation of lipid droplets was observed in rabeprazole-treated CT26 cells (Fig. 7b). To evaluate the antitumor effect of rabeprazole in vivo, we established colon cancer transplanted tumor models by injecting CT26 cells into BALB/c mice. Rabeprazole treatment significantly suppressed the tumor growth in mice as measured by tumor volume (Fig. 7c). Additionally, upregulation of CD8 and downregulation of PD1 was detected by immunohistochemical staining (Fig. 7e), confirming that an antitumor immune response was stimulated by rabeprazole. Furthermore, we investigated the effect of rabeprazole on lipid droplet accumulation and tumor growth after ARF1 knockdown to prove that the anti-tumor effect of rabeprazole is ARF1 dependent.
Off-target screening
RNAi-based drug design: considerations and future directions - Nature.com
RNAi-based drug design: considerations and future directions.
Posted: Wed, 03 Apr 2024 07:00:00 GMT [source]
The extracted sequence and structural features of drugs and targets were then input into a hybrid fusion module to comprehensively integrate and fuse the interaction information between drugs and targets. Subsequently, the fused features were fed into a FC network to predict drug-target affinity. Although deep learning-based methods for drug-target interaction, affinity, and binding sites identification have provided complete datasets and codes, only a few have made online platforms available for biologists and pharmacists to use [30, 31]. However, it can be challenging for biologists and pharmacists to reproduce these codes and run them on the provided datasets. The design algorithm has demonstrated its capability to successfully generate molecules with desired properties by incorporating an additional encoding within the input. This encoding allows for the translation of various drug discovery-relevant properties with high accuracy into the generated molecules.
Assessing sequence-based protein–protein interaction predictors for use in therapeutic peptide engineering
As described in the communication entitled “Cytotoxicity of Different Excipients on RPMI 2650 Human Nasal Epithelial Cells” [11], they found that all additives at 0.3% sodium hyaluronate and polyvinyl alcohol at 1% concentrations can be safely used for nasal formulations. 3-D QSAutogrid-R MPGRS example image On June 18, 2011 the CoMFA patent has dropped any restriction on the use of GRID and PLS technologies and the RCMD team (rcmd.it) has opened a 3D QSAR web server (3d-qsar.com) based on the 3-D QSAutogrid/R engine. 3-D QSAutogrid/R covers all the main features of CoMFA and GRID/GOLPE with implementation by multiprobe/multiregion variable selection (MPGRS) that improves the simplification of interpretation of the 3-D QSAR map. The methodology is based on the integration of the molecular interaction fields as calculated by AutoGrid and the R statistical environment that can be easily coupled with many free graphical molecular interfaces such as UCSF-Chimera, AutoDock Tools, JMol and others. Artificial intelligence (AI) is having an impact on every aspect of our lives – research included. This aims to position Switzerland as a leading global location in which to develop and use transparent and trustworthy AI.
Current Methods for Drug Design
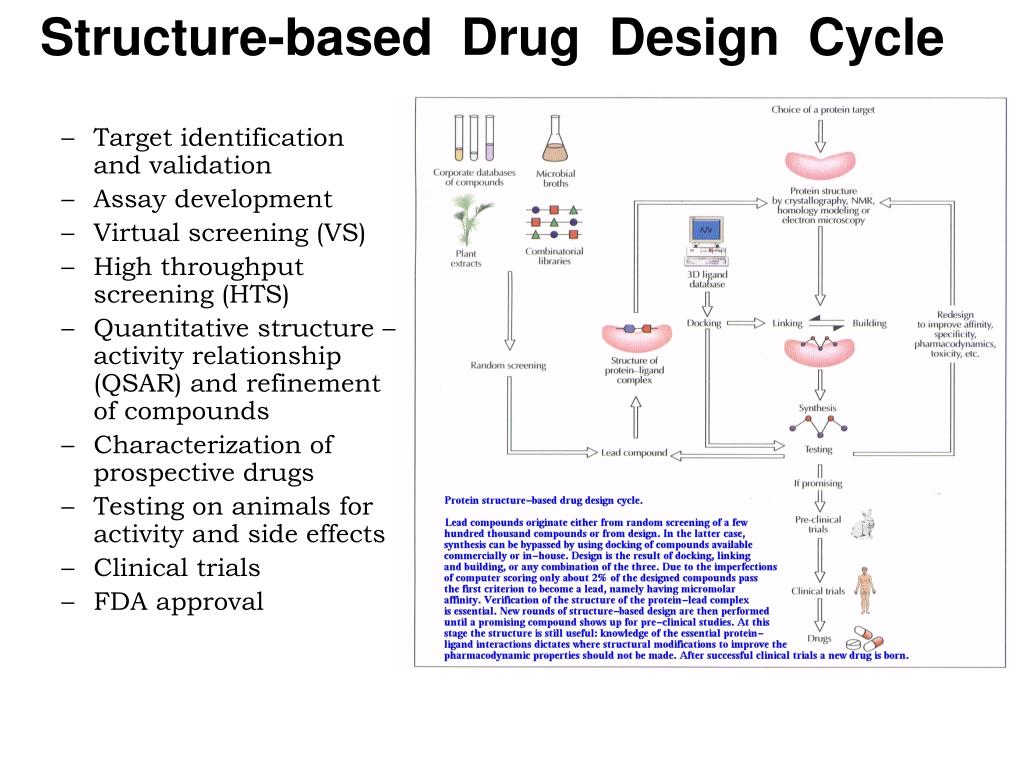
We checked this dataset with our training set and found only 1,062 pairs (5.8%) were overlapped. The majority of this dataset are not seen by TransformerCPI2.0 and baseline models during the training phase, so it can measure the generalization of these models to some extent. However, this analysis does not prove that TranformerCPI2.0 can solve activity cliff prediction problems. We stress that this analysis is aim to show that TransformerCPI2.0 can capture activity-related information of compounds, and can served as interpretation tools.
One network processed drug-target binding pocket graphs, while the other handled 2D drug molecule graphs. Secondly, for deep learning-based drug-target affinity prediction methods, both DeepDTA [21] and DeepDTAF [22] used 1D-CNN to extract sequence features from drugs and targets for predicting drug-target affinity. GraphDTA [23], SAG-DTA [24], GraphCL-DTA [25], TDGraphDTA [26], and other related methods employed GNN to extract the structural features of drug molecules. Meanwhile, natural language processing models such as CNN and RNN were utilized to extract sequence features. Furthermore, method proposed by [27] and MMDTA [28] simultaneously made use of both the sequence and structural features of drug and target.
Second, we used case studies to interpret our model to verify whether it learns knowledge as expected, rather than exhibiting only data bias20. Third, we applied TransformerCPI2.0 to discover new hits for challenging targets, speckle-type POZ protein (SPOP) and ring finger protein 130 (RNF130), which lacks existing 3D structures. Additionally, we identified ADP-ribosylation factor 1 (ARF1) as a new target for proton pump inhibitors (PPIs).
No comments:
Post a Comment